Zink et al. 2021 provides the first full K2 transiting exoplanet sample, using photometry from Campaigns 1-8 and 10-18, derived through a fully automated procedure. To encourage transparency and to benefit the community, I provide the following data products:
Citations:
If you make use of any of these products, please be sure to cite Zink et al. (2020; 2021) accordingly.
Citations:
If you make use of any of these products, please be sure to cite Zink et al. (2020; 2021) accordingly.
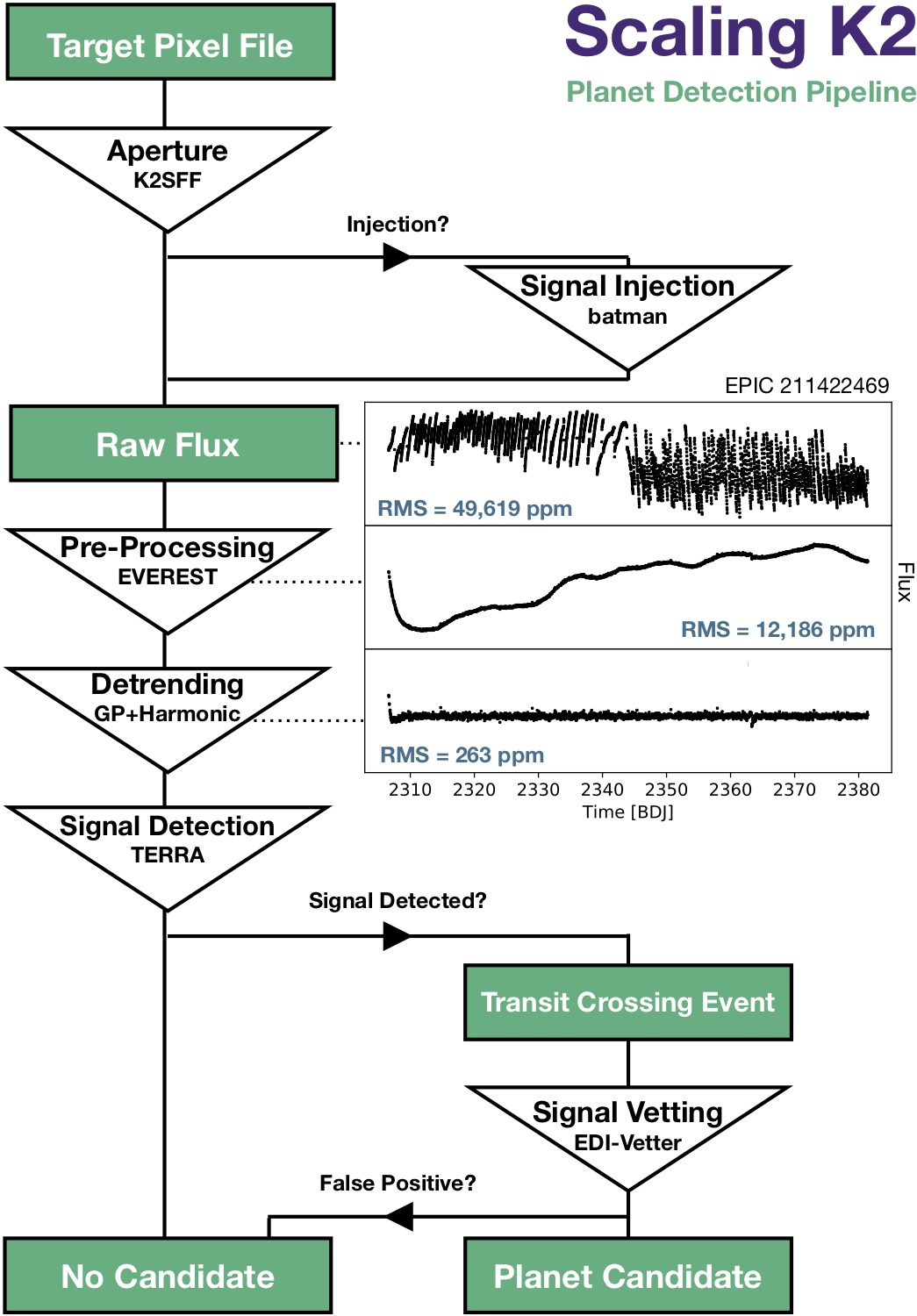
Our automated pipeline relies on three major software components:
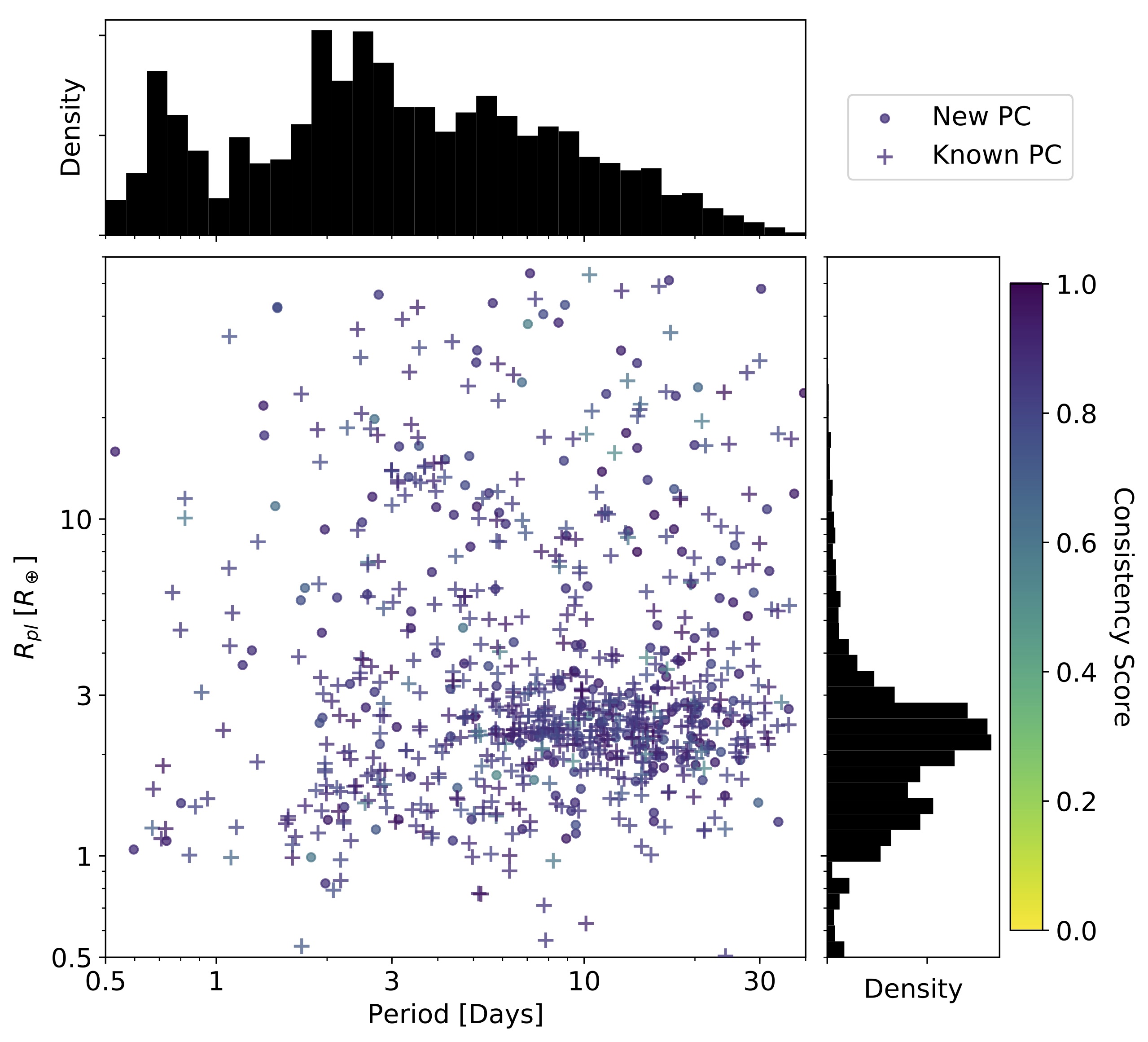
Using our fully automated pipeline, we achieve a sample of K2 transiting planets suitable for demographics. Within this catalog we find:
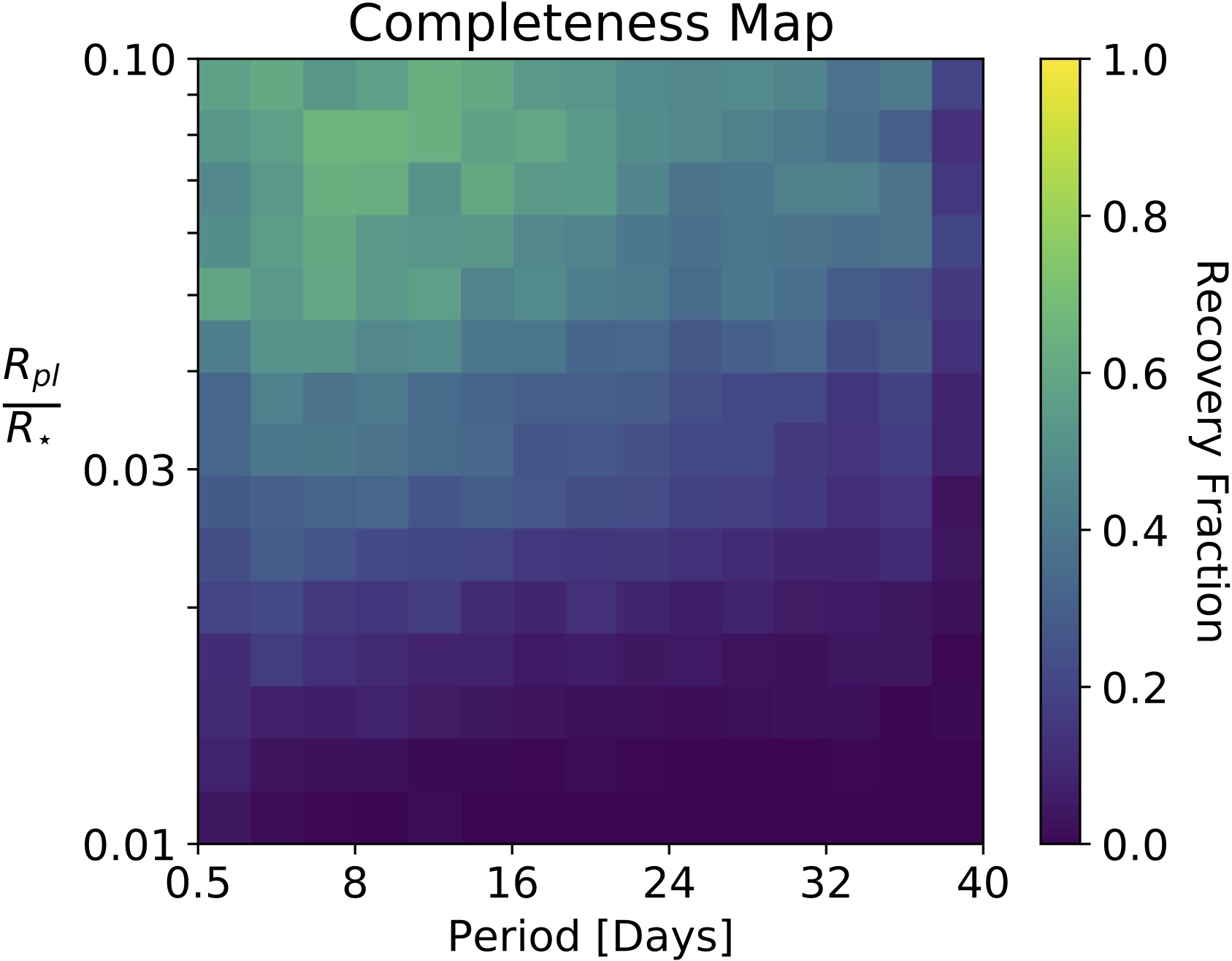
Any catalog of planets will inherit some selection effects due to the methodology of detection, limitations of the instrument, and stellar noise. These biases will effect the sample completeness and must be accounted for when conducting a demographic analysis. The selection of transiting planets can be addressed using analytic arguments, but the instrument and stellar noise contributions to the sample completeness depend on the stellar sample and the specifics of the instrument. With an automated detection pipeline, this detection efficiency mapping can be achieved through the implementation of an injection/recovery test. Here, artificial signals are injected into the raw photometry and run through the automated software to test the pipeline’s recovery capabilities, directly measuring the impact of instrument and stellar noise on the catalog. Here we provide the necessary data products to make such measurements. ________________________________________________________ Light Curve Noise Measure: To assess the noise properties within each detreneded light curve, we measure the combined differential photometric precision (CDPP; Christiansen et al. 2012) for transit duration of 1, 1.5, 2, 2.5, 3, 4, 5, 6, 7, 8, 9, and 10 hours. These values enable users to analytically calculate the injected signal strength. The corresponding values are provide in an ASCII file.
|
|
|
|
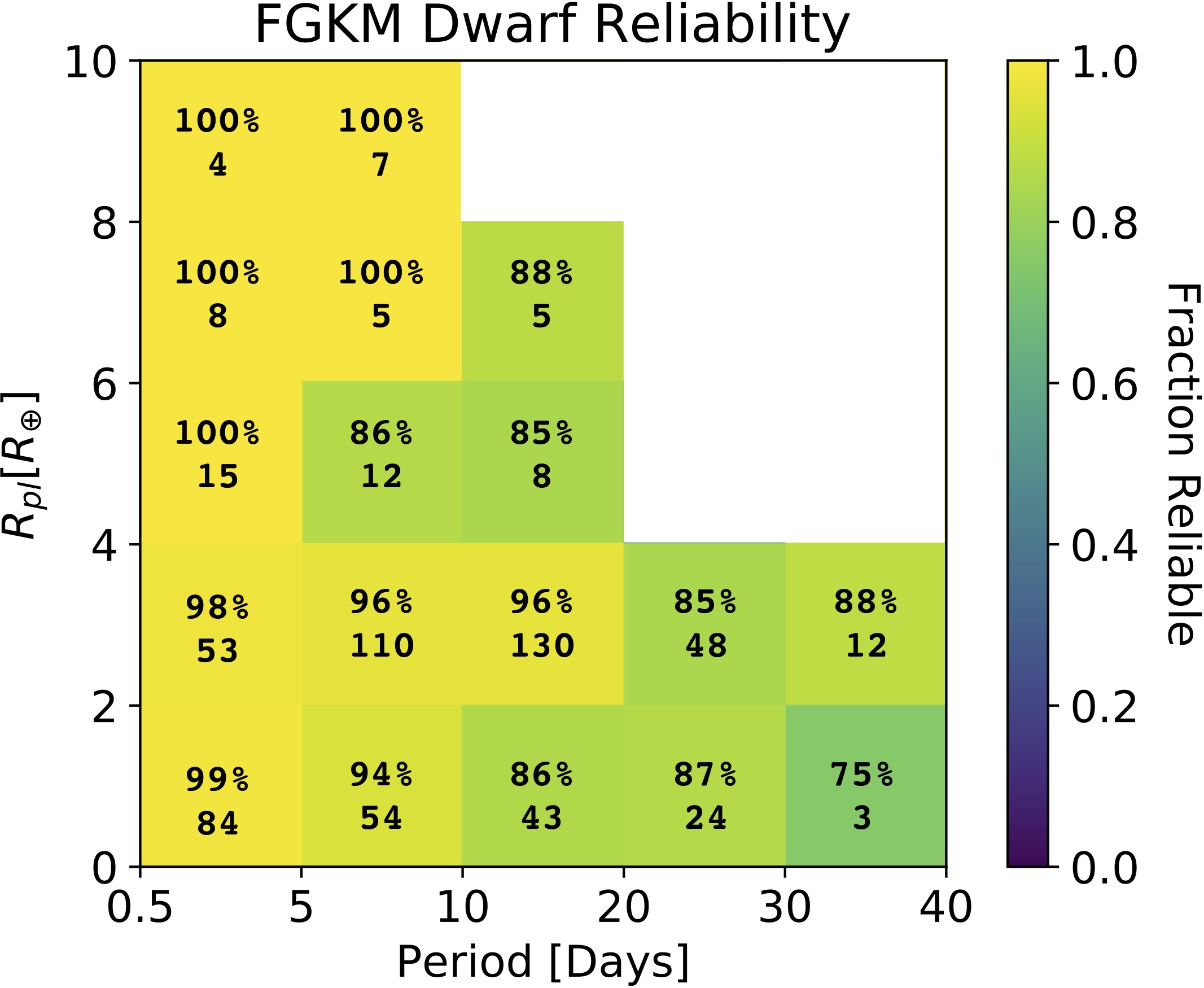
Despite efforts to remove problematic cadences, instrument systematics pollute the light curve data, creating artificial dips that can be erroneously characterized as a transit signal. To measure the purity in a homogeneous catalog of planets, the rate of these false alarms (FAs) must be quantified.
The main goal of EDI-Vetter is to parse through all TCEs and remove FAs without eliminating true planet candidates. However, this process is difficult to automate and requires a method of testing the software's capability to achieve this goal. Accomplishing such a task necessitates an equivalent data set that captures all unique noise properties that contribute to FAs, without the existence of any true astrophysical signals. With such data available, the light curves can be processed through the detection pipeline. By inverting the light curves and re-running our analysis, we achieve such a simulation and provide the resulting FAs in the following CSV file: